
- BUILDING RANDOM COPOLYMER IN MATERIALS STUDIO MANUAL
- BUILDING RANDOM COPOLYMER IN MATERIALS STUDIO VERIFICATION
- BUILDING RANDOM COPOLYMER IN MATERIALS STUDIO TRIAL
BUILDING RANDOM COPOLYMER IN MATERIALS STUDIO MANUAL
1, power conversion efficiency (PCE) can only be recognized after manual designing, material engineering, and devices engineering.
BUILDING RANDOM COPOLYMER IN MATERIALS STUDIO TRIAL
Clearly, the consumption on trial and error experiments is huge. It mainly results from failed design strategies of D/A materials and/or unsuitable synergistic effects of D/A combinations. Apart from materials reported in these publications, there are imponderable materials, which have been synthesized and characterized while they have not been reported ever owing to low performance in OSC devices. More than 60% of these publications are based on material science, mainly involved in material design for photoactive layers in OSCs. More than 50,000 publications focusing on OSCs have been tracked by Web of Science (see Supplementary Fig. In this process, tens of thousands of photovoltaic materials have been designed and synthesized by chemists and materials scientists. In the latest decade, the discovery of novel photoactive donor (D) and acceptor (A) materials has greatly promoted the development of bulk heterojunction (BHJ) organic solar cells (OSCs) 1, 2, 3, 4, 5, 6, 7.
BUILDING RANDOM COPOLYMER IN MATERIALS STUDIO VERIFICATION
The outcome of ML and experiment verification demonstrates that the RF approach can be effectively applied to high-throughput virtual screening for opening new perspectives to design of materials and D/A pairs, thereby accelerating the development of OSCs. Among them, six photovoltaic D/A pairs are selected and synthesized to compare their predicted and experimental power conversion efficiencies. Furthermore, >32 million D/A pairs were screened and calculated by RF and BRT models, respectively. Thus, the best predictive capabilities are provided by using the random forest (RF) and boosted regression trees (BRT) approaches beyond other ML algorithms in the data set. In this study, we constructed five common algorithms, and introduced 565 donor/acceptor (D/A) combinations as training data sets to evaluate the practicalities of these ML algorithms and their application potential when guiding material design and D/A pairs screening. Yet, like model selection in statistics, the choice of appropriate machine learning (ML) algorithms plays a vital role in the process of new material discovery in databases.
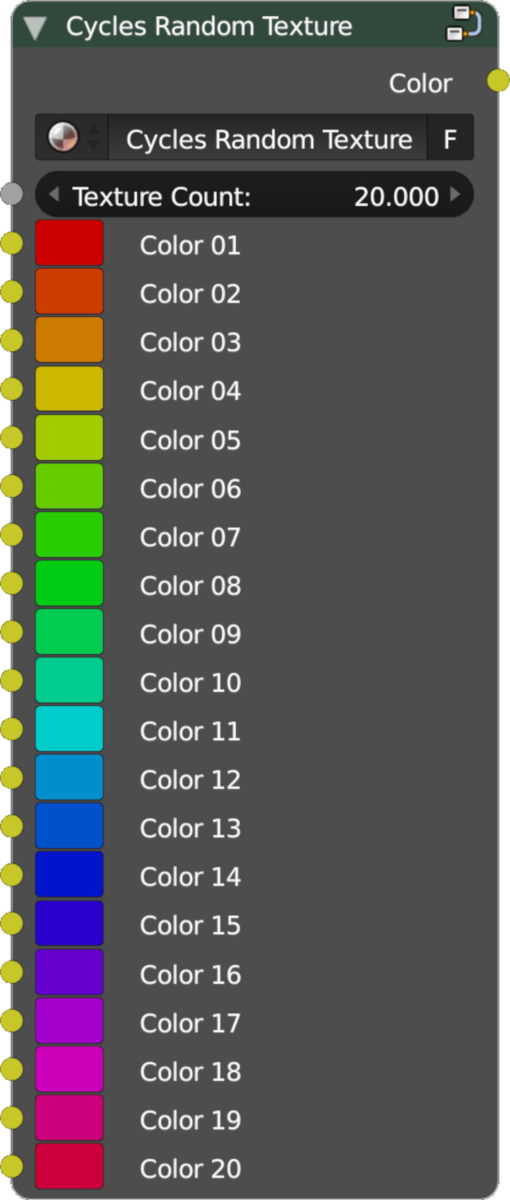

Integrating artificial intelligence (AI) and computer science together with current approaches in material synthesis and optimization will act as an effective approach for speeding up the discovery of high-performance photoactive materials in organic solar cells (OSCs).
